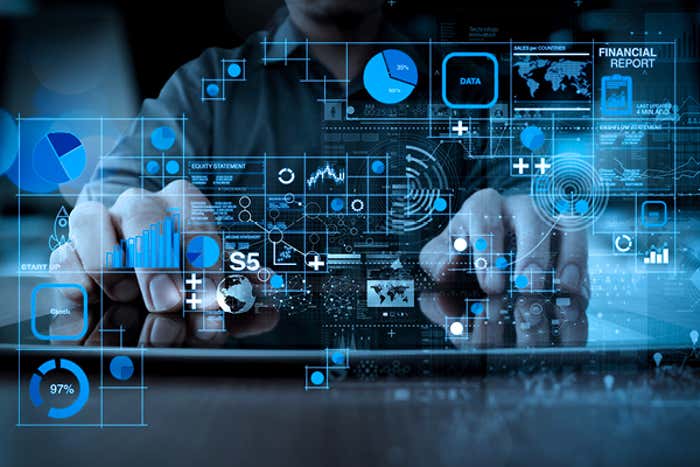
In 2023, generative AI took center stage—the year was filled with rapid advancements in the capabilities of large language models (LLMs). Heading into 2024, businesses continue to see great potential for generative AI and are eager to integrate the technology into internal workflows and customer-facing applications.
Developers are engaging in a variety of techniques to improve the performance of LLMs for domain-specific tasks. At times, these efforts have been loosely referred to as “fine tuning.” True “fine tuning” involves the further training of an LLM with new data (i.e., the “re-weighting” of the LLM’s parameters). However, “fine tuning” is at times overused to describe techniques that do not involve updating an LLM’s weights.
Fine tuning can be time- and resource-intensive. As a result, developers often prefer to tailor LLMs for domain-specific tasks using prompt engineering. As the name suggests, prompt engineering consists of crafting high-quality inputs.
Retrieval-augmented generation (RAG) is a prompt engineering technique that consists of an intermediary step between a user’s submission of a prompt and the LLM’s generation of output. In this step, an LLM-based application retrieves additional information of greater relevance and higher quality that was not part of its training, such as:
- recent data from search engine results (e.g., ChatGPT’s “Browse with Bing” functionality) and
proprietary datasets (e.g., a custom GPT that retrieves from an enterprise’s knowledge base).
The user’s original prompt is then augmented with the retrieved information and provided to the LLM. As a result, a RAG-enabled application may generate more relevant outputs, and potentially fewer hallucinations, without any retraining.
We anticipate that the adoption of RAG will continue to grow in 2024. When assessing the suitability of a RAG-enabled application for a given use case, businesses should consider the sources from which the application retrieves data.